Forward Dynamic Simulations of Pitching Mechanics
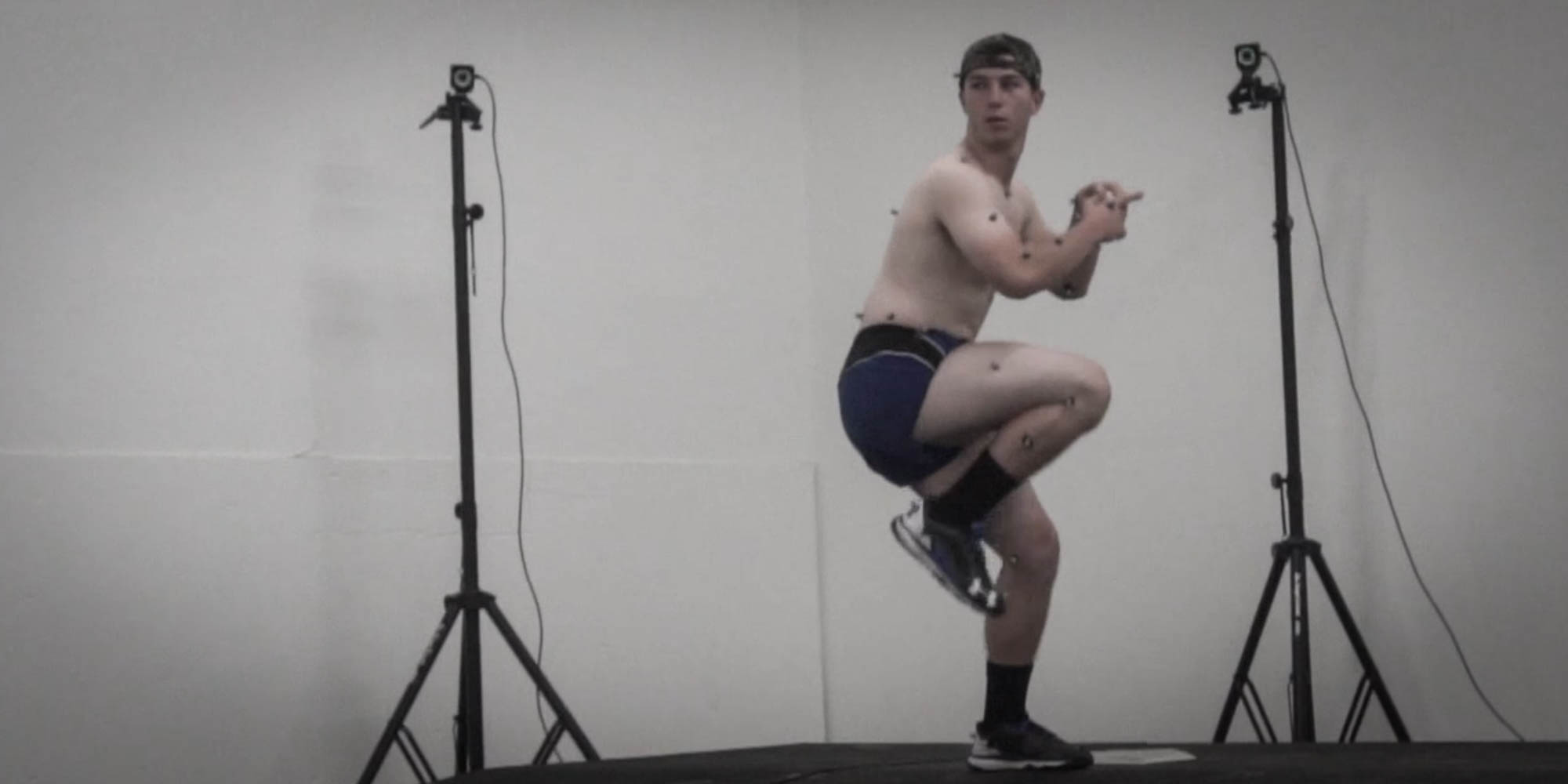
Previously at Driveline Baseball, we featured an exciting and informative three part series by Dr. James Buffi on his work toward an innovative new training and elbow injury prevention approach that has the potential to better protect a pitcher’s weak link- the UCL (Part One). While traditional methods have analyzed the pitching motion using an Inverse approach, calculating forces and torques based on observed joint angles and positions (Part Two), Dr. Buffi established a Forward Dynamic approach that can more precisely determine individual muscle contributions toward protecting the UCL using a novel simulation framework (Part Three).
(This article was written by Matthew Wagshol, Biomechanist in the R&D Department of Driveline Baseball.)
Driveline Baseball R&D has now validated this simulation approach and in this new three-part series, we will reveal the inner workings of the modeling process, discuss new insights from the resulting data analysis, and then look at further experimentation and case studies that will improve applications of the model to pitching training protocols.
(Note from Kyle Boddy, Director of R&D: It’s hard for me to overstate how exciting this years-long project is and how important it can be to baseball. It represents a completely new way of modeling the pitching motion by analyzing soft tissue and computed muscle outputs to get a “forward” looking method developed, which could lead to far more granular ways to test and train baseball pitchers.)
Let’s begin by diving into the peer reviewed, open source pitching simulation pipeline from Dr. Buffi’s dissertation (1) and gain a detailed understanding of what it takes to produce an accurate Forward Dynamics model.
- Generalized Forward Dynamics Simulation Pipeline (6)
Motion Capture
Before a simulation can begin, we have to capture the motion of a pitch. This is generally performed using a marker based motion capture system of high speed cameras and force plates. For our validation of the Forward Dynamics model, we used the same ideal pitching case as Dr. Buffi, from a seperate 2008 paper (2) in order to compare our model and validate its accuracy. In this study, the motion was captured using a 7-camera Vicon 612 motion capture system and three AMTI force platforms.
Model Modification
The recorded experimental marker data is then mapped onto an open source model of human body biomechanics using the OpenSIM platform that is updated specifically for studying the elbow during the pitching motion. This is accomplished through modifying a standard 50th percentile male full body model by replacing the right arm with a refined upper arm muscle model (3). Other adjustments to the model were made to better suit it to pitching analysis, including adding a Varus-Valgus DOF at the elbow (4), focusing on the 14 medial muscle compartments that can produce a varus torque to protect the UCL, and simplifying the Flexor Digitorum Superficialis’ four sub compartments to all begin at the same insertion point.
Scaling
- Scaled Model on Left- taller and more mass, muscles in blue and red, virtual markers pink
In addition to capturing a pitching motion, the subject is also filmed in a static standing pose allowing us to scale the generic model to an individual pitcher. By placing virtual markers on the model in the same locations as in the recorded trial, the segments of the body are linearly scaled to match an individual body’s actual dimensions. This process also updates the mass of each body segment to align with the subject’s new total mass, as well as adjusting the muscle paths and lengths.
Inverse Kinematics
With a scaled model, we are now ready to initially track the recorded motion and recreate the associated joint angles and accelerations through a traditional Inverse Kinematics approach. Markers are weighted to best reproduce the overall motion and time frames are noted for key events during the pitch with a focus on the range of highest valgus torque from arm cocking through maximum external rotation to release (5).
- Notes: Dashed line is time of peak Valgus torque, elbow is flexing, just prior to MER
- Varus-Valgus DOF is locked since only very small laxity at the joint
By completing an inverse kinematic analysis we are able to confirm that the pitching motion has been accurately reproduced, and also provide the core marker trajectories that a forward dynamic, muscle-based analysis will attempt to track.
Inverse Dynamics
We also produce a traditional Inverse Dynamics analysis that calculates general forces and torques from the motion kinematics in order to confirm the specific instant of peak elbow valgus torque and its magnitude.
- Comparison of imposed Valgus torque in model (top) with Fleisig paper (bottom)
In our simulation, the peak valgus load is measured to be approximately 120 Nm at 6.33s, which occurs just prior to MER at 6.35s. The motion is filtered at different frequencies to remove noise, with 30hz used for this study to gain a precise look at the torques acting on the elbow.
Residual Reduction Analysis
One final adjustment is then made to the model before we are ready to initiate the Forward Dynamics analysis. In order to reduce experimental errors and ensure the accuracy of the updated masses from the initial scaling step, the simulation is run with only ideal torque actuators as well as inputs from the ground reaction forces recorded by the force plates. This step uses a Residual Reduction Algorithm to make small necessary adjustments to the model bodies’ masses as well as to the overall center of mass to obtain dynamic consistency with the measured ground reaction forces. When these adjustments are a small portion (under 2%) of the model’s total mass, we can confirm that the model, its motion, and the ground forces are linked and accurately representing the recorded trial.
—
We are now ready to begin a Forward Dynamic analysis using OpenSIM’s Computed Muscle Control! In the next blog post of this series, we will explore the CMC process and look at the results of individual muscle contributions, the associated joint compression force, and the total load on the UCL as we continue with the validation of our simulation framework.
Sources:
1) James H. Buffi, Katie Werner, Tom Kepple, and Wendy M. Murray, Computing muscle, ligament, and osseous contributions to the elbow varus moment during baseball pitching Ann Biomed Eng. 2015 Feb;43(2):404-15
2)Alderink, A., T. Kepple, K. L. Siegel, A. Razzook, and S. Stanhope. Sources of forward ball velocity in a pitched baseball. Paper presented at 32nd Annual Meeting of the American Society of Biomechanics 2008; Ann-Arbor, MI.
3)Saul, K. R., X. Hu, C. M. Goehler, et al. Benchmarking of dynamic simulation predictions in two software platforms using an upper limb musculoskeletal model. Comput. Methods Biomech. Biomed. Eng. 2014. doi:10.1080/1025 5842.2014.916698.
4)Buchanan, T. S., S. L. Delp, and J. A. Solbeck. Muscular resistance to varus and valgus loads at the elbow. J. Biomech. Eng.-T. ASME 120:634–639, 1998
5)Glenn S Fleisig, Rafael F Escamilla, Biomechanics of the elbow in the throwing athlete, Operative Techniques in Sports Medicine 4(2):62-68 · April 1996
6)Thelen, D. G., F. C. Anderson, and S. L. Delp. Generating dynamic simulations of movement using computed muscle control. J. Biomech. 36:321–328, 2003.
Comment section
Add a Comment
You must be logged in to post a comment.
Computed Muscle Control Analysis of Pitching Mechanics -
[…] with our validation of a Forward Dynamic analysis of the pitching motion from last week (Part One), we had run through the initial stages of the simulation pipeline to have an accurately scaled […]